Automatic segmentation of femoral tumors by nnU-net
Clinical Biomechanics(2024)
摘要
Background
Metastatic femoral tumors may lead to pathological fractures during daily activities. A CT-based finite element analysis of a patient's femurs was shown to assist orthopedic surgeons in making informed decisions about the risk of fracture and the need for a prophylactic fixation. Improving the accuracy of such analyses ruires an automatic and accurate segmentation of the tumors and their automatic inclusion in the finite element model. We present herein a deep learning algorithm (nnU-Net) to automatically segment lytic tumors within the femur.
Method
A dataset consisting of fifty CT scans of patients with manually annotated femoral tumors was created. Forty of them, chosen randomly, were used for training the nnU-Net, while the remaining ten CT scans were used for testing. The deep learning model's performance was compared to two experienced radiologists.
Findings
The proposed algorithm outperformed the current state-of-the-art solutions, achieving dice similarity scores of 0.67 and 0.68 on the test data when compared to two experienced radiologists, while the dice similarity score for inter-individual variability between the radiologists was 0.73.
Interpretation
The automatic algorithm may segment lytic femoral tumors in CT scans as accurately as experienced radiologists with similar dice similarity scores. The influence of the realistic tumors inclusion in an autonomous finite element algorithm is presented in (Rachmil et al., 2024 [14]).
更多查看译文
关键词
nnU-net,Femur,Segmentation
AI 理解论文
溯源树
样例
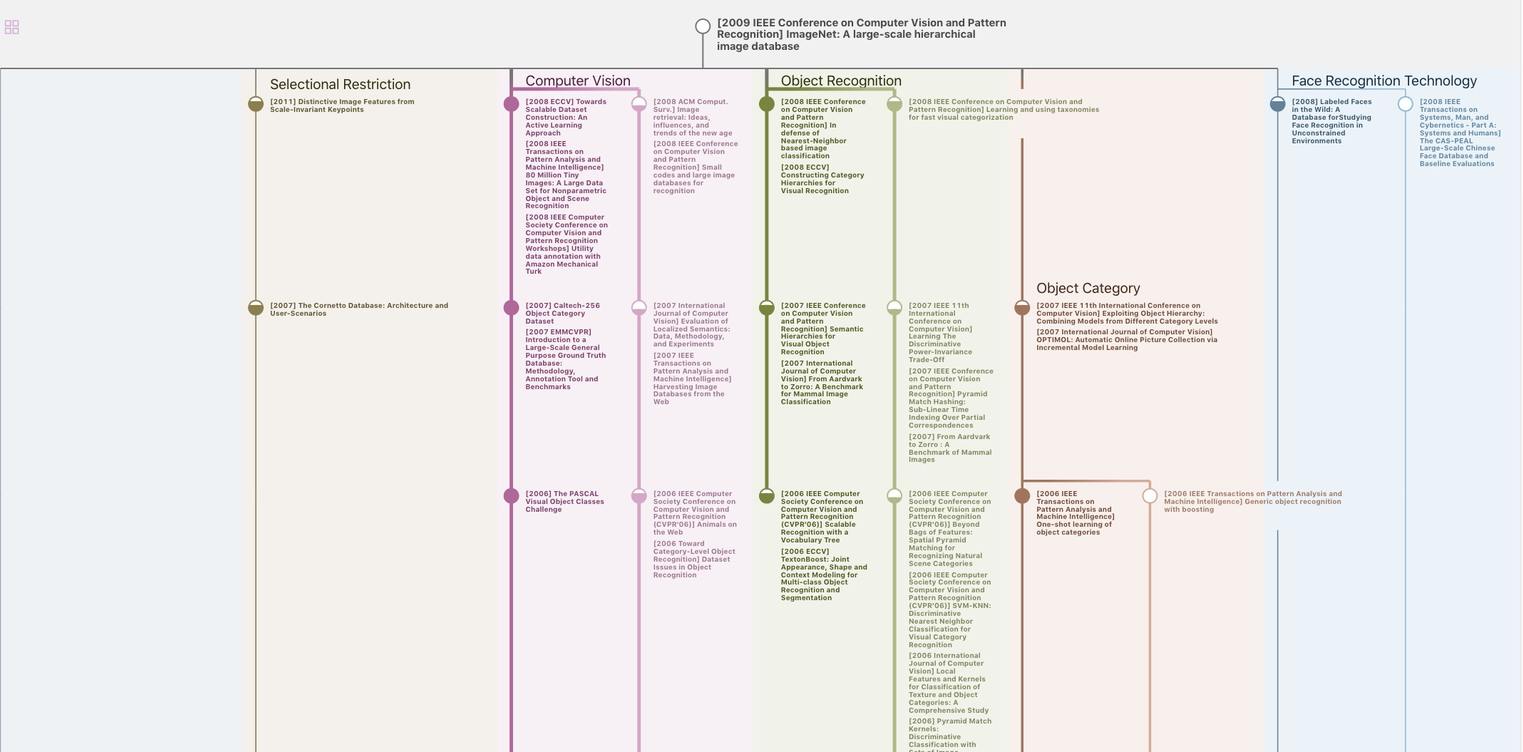
生成溯源树,研究论文发展脉络
Chat Paper
正在生成论文摘要