Deep learning to detect left ventricular structural abnormalities in chest X-rays
EUROPEAN HEART JOURNAL(2024)
摘要
Background and Aims Early identification of cardiac structural abnormalities indicative of heart failure is crucial to improving patient outcomes. Chest X-rays (CXRs) are routinely conducted on a broad population of patients, presenting an opportunity to build scalable screening tools for structural abnormalities indicative of Stage B or worse heart failure with deep learning methods. In this study, a model was developed to identify severe left ventricular hypertrophy (SLVH) and dilated left ventricle (DLV) using CXRs.Methods A total of 71 589 unique CXRs from 24 689 different patients completed within 1 year of echocardiograms were identified. Labels for SLVH, DLV, and a composite label indicating the presence of either were extracted from echocardiograms. A deep learning model was developed and evaluated using area under the receiver operating characteristic curve (AUROC). Performance was additionally validated on 8003 CXRs from an external site and compared against visual assessment by 15 board-certified radiologists.Results The model yielded an AUROC of 0.79 (0.76-0.81) for SLVH, 0.80 (0.77-0.84) for DLV, and 0.80 (0.78-0.83) for the composite label, with similar performance on an external data set. The model outperformed all 15 individual radiologists for predicting the composite label and achieved a sensitivity of 71% vs. 66% against the consensus vote across all radiologists at a fixed specificity of 73%.Conclusions Deep learning analysis of CXRs can accurately detect the presence of certain structural abnormalities and may be useful in early identification of patients with LV hypertrophy and dilation. As a resource to promote further innovation, 71 589 CXRs with adjoining echocardiographic labels have been made publicly available. Structured Graphical Abstract The deep learning model that was developed takes as input a pre-processed chest X-ray of dimension 224-by-224, age, and sex; the model outputs a probability for dilated left ventricle, severe left ventricular hypertrophy, and a composite label indicating the presence of either structural abnormality. The model outperforms all 15 board-certified radiologists in the task of detecting the presence of either abnormality (composite label). For a single point of comparison, we used the consensus vote amongst radiologists. The model achieves a sensitivity of 71% compared with the consensus vote sensitivity of 66% at a fixed specificity of 73%. Saliency maps demonstrate that at shallower layers in the network, the model is sensitive to the broader cardiac silhouette as well as structures in the left heart. AI, artificial intelligence; AUROC, area under the receiver operating characteristic curve; CXR, chest X-ray; DLV, dilated left ventricle; IVSd, interventricular septal thickness at end-diastole; LVIDd, left ventricular internal diameter at end-diastole; LVPWd, left ventricular posterior wall distance at end-diastole; SLVH, severe left ventricular hypertrophy.
更多查看译文
关键词
Deep learning,Chest X-rays,Early detection,Heart failure,Dilated left ventricle,Left ventricular hypertrophy
AI 理解论文
溯源树
样例
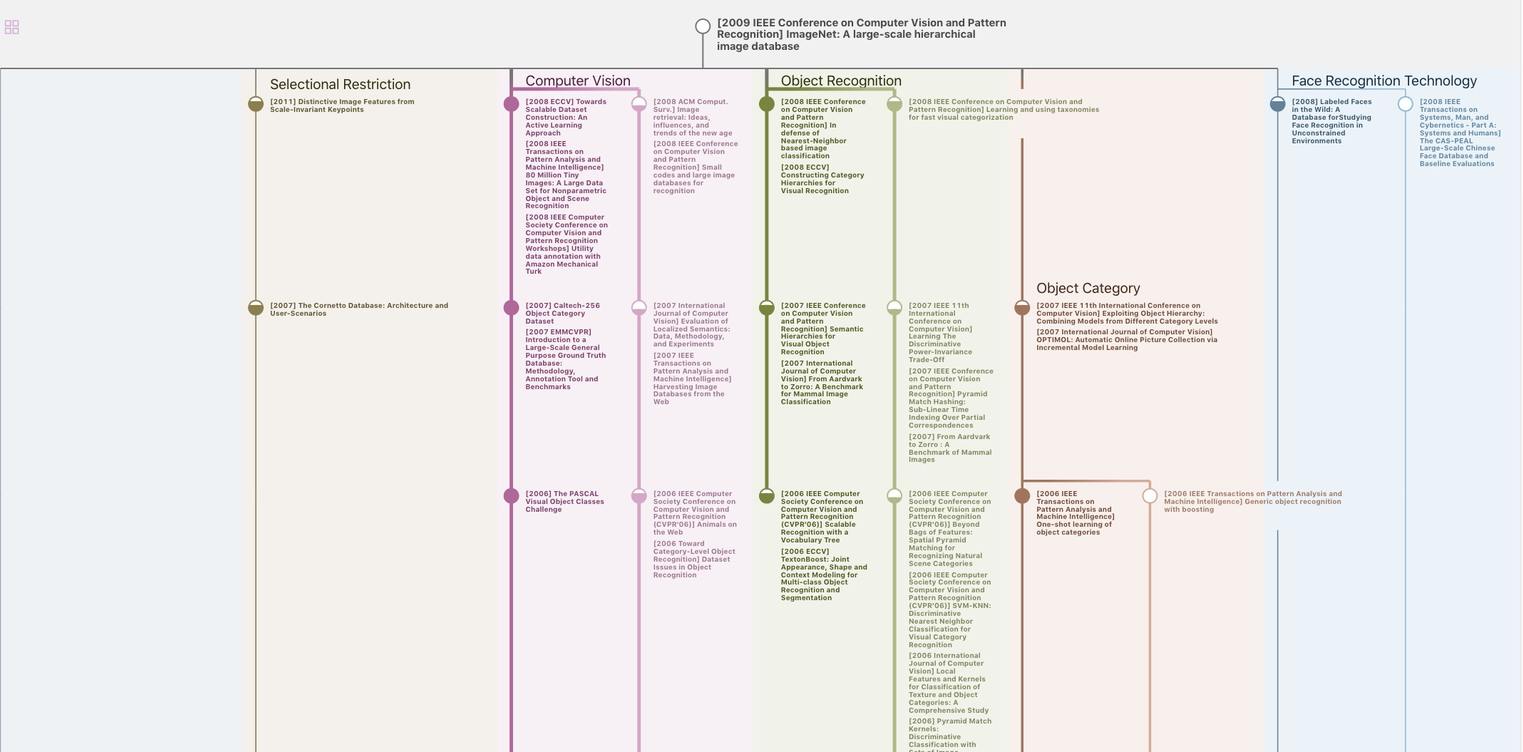
生成溯源树,研究论文发展脉络
Chat Paper
正在生成论文摘要