Confidence intervals of survival predictions with neural networks trained on molecular data
Informatics in Medicine Unlocked(2024)
摘要
In medicine, an important objective is predicting patients’ survival based on their molecular and clinical characteristics. In this context, neural networks have recently been used for their ability to capture complex interactions in the data. Measuring the uncertainty associated with survival estimates obtained by neural networks is essential to enhance predictions’ reliability. We compared four methods adapted to multilayer perceptrons (MLPs) for building confidence intervals at the patient level. The methods were based either on bootstrap with Boot (Efron, 1979), ensembling with DeepEns (Lakshminarayanan et al., 2016), or Monte-Carlo Dropout with MCDrop and BMask (Gal and Ghahramani, 2016; Mancini et al., 2020). A comparison was made through MLP-based survival models: CoxCC and CoxTime (Kvamme et al., 2019) in a continuous time framework, DeepHit (Lee et al., 2018) and PLANN (Biganzoli et al., 1998) in a discrete time framework. We applied the methods to a simulation study, enabling us to estimate a coverage rate of the estimated confidence intervals. We also applied them to real-world datasets, and predicted the survival probability for patients with breast cancer and patients with lung cancer.
更多查看译文
关键词
Machine learning,Multi-layer perceptrons,Confidence intervals,Uncertainty,Survival prediction
AI 理解论文
溯源树
样例
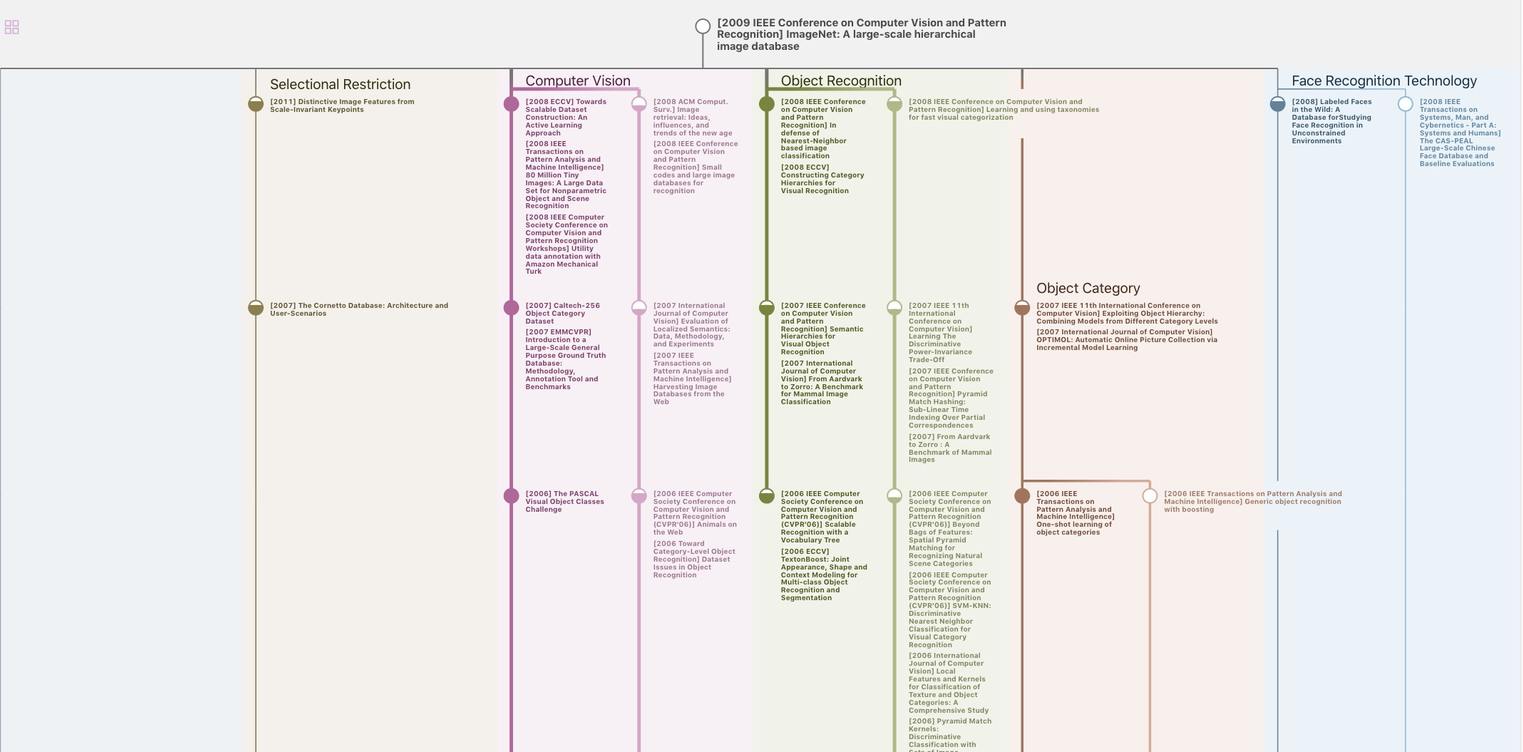
生成溯源树,研究论文发展脉络
Chat Paper
正在生成论文摘要