P-621 Machine learning software significantly increase clinical pregnancy rates in natural frozen-thawed embryo transfer cycles
Human Reproduction(2023)
摘要
Study question To study the effect of physician support software for determining ovulation time on pregnancy outcomes in natural cycle frozen embryo transfers (NC-FET) Summary answer Match between the physician and the algorithm regarding the time of ovulation in NC-FET cycles resulted in significant 14.4% increase in clinical pregnancy rates. What is known already Today the preferred method for frozen embryo transfer is natural cycle based on ovulation detection. As of today, an algorithm for predicting ovulation has not been developed and its use and its effect on success rates have not been tested. The aim of this study was to test a physician support artificial intelligence (AI) software for determining ovulation time and to study the effect of its use on pregnancy outcomes in frozen-thawed embryo transfers (NC-FET). Study design, size, duration NC-FET cycles from September 2018 to June 2021 were used to develop ovulation detection algorithm. Next, the effect of the selection of the ovulation date by the algorithm on pregnancy rates was tested by retrospective analysis on NC-FET cycles performed between July 2021 to June 2022. Cycles were divided into two groups: The Matched group in which there was a match between the ovulation date set by the physician and the algorithm and Mismatched group. Participants/materials, setting, methods Study group included 349 single embryo transfer patients after applying strict inclusion criteria. The Matched group included 198 patients and 151 patients in the Mismatched group. Patient's age, BMI, smoking, number of past retrievals and past embryo transfers, endometrium thickness, embryos age and quality, number of tests per cycle and pregnancy rates were compared. Statistical analysis included multivariable logistic regression to study the association between Match/Mismatch and clinical pregnancy rates while controlling for potential confounders Main results and the role of chance Matched group and Mismatched group had similar characteristics. Mean patients age at oocyte retrieval were 34.5 + 5.5 vs. 34.7 + 5.5 respectively (p=0.72). Clinical pregnancy rate in the Matched group were 38.9% compared to 24.4% in the Mismatched group (p =0.006). The difference was particularly noticeable when day 3 embryos were transferred (39.8% vs. 17.6% respectively p=0.009), and was also observed on day 5 embryos transfer, although non-significant (39.1% vs. 30.0% respectively, p=0.197). In the Matched group the chance of pregnancy actually almost doubled (OR = 1.97, CI 95% 1.23-3.17, p=0.005) while controlling potential confounders. Further analysis of the Mismatch group found that in 120 cycles (%) the doctor set the ovulation date one day or more before the date set by the algorithm and in 31(%) cases the date was a day or more after the ovulation date according to the algorithm. Logistic regression showed that the chance of pregnancy was significantly affected when the doctor determines the day of ovulation earlier than the algorithm (OR = 0.42, CI 95% 0.29-1.43, p=0.005) Limitations, reasons for caution Although cycles selected for comparison were controlled for key factors that affect pregnancy outcomes, The main drawback is the retrospective analysis. Further prospective randomized trials are needed to study the effectiveness of the algorithm and its effect on treatment results. Wider implications of the findings This is the first AI algorithm designed to predict ovulation. Using AI algorithms in NC-FET can help in identifying ovulation accurately and reliably and may increase pregnancy rate Trial registration number Not applicable
更多查看译文
关键词
embryo transfer cycles,clinical pregnancy rates,machine learning,frozen-thawed
AI 理解论文
溯源树
样例
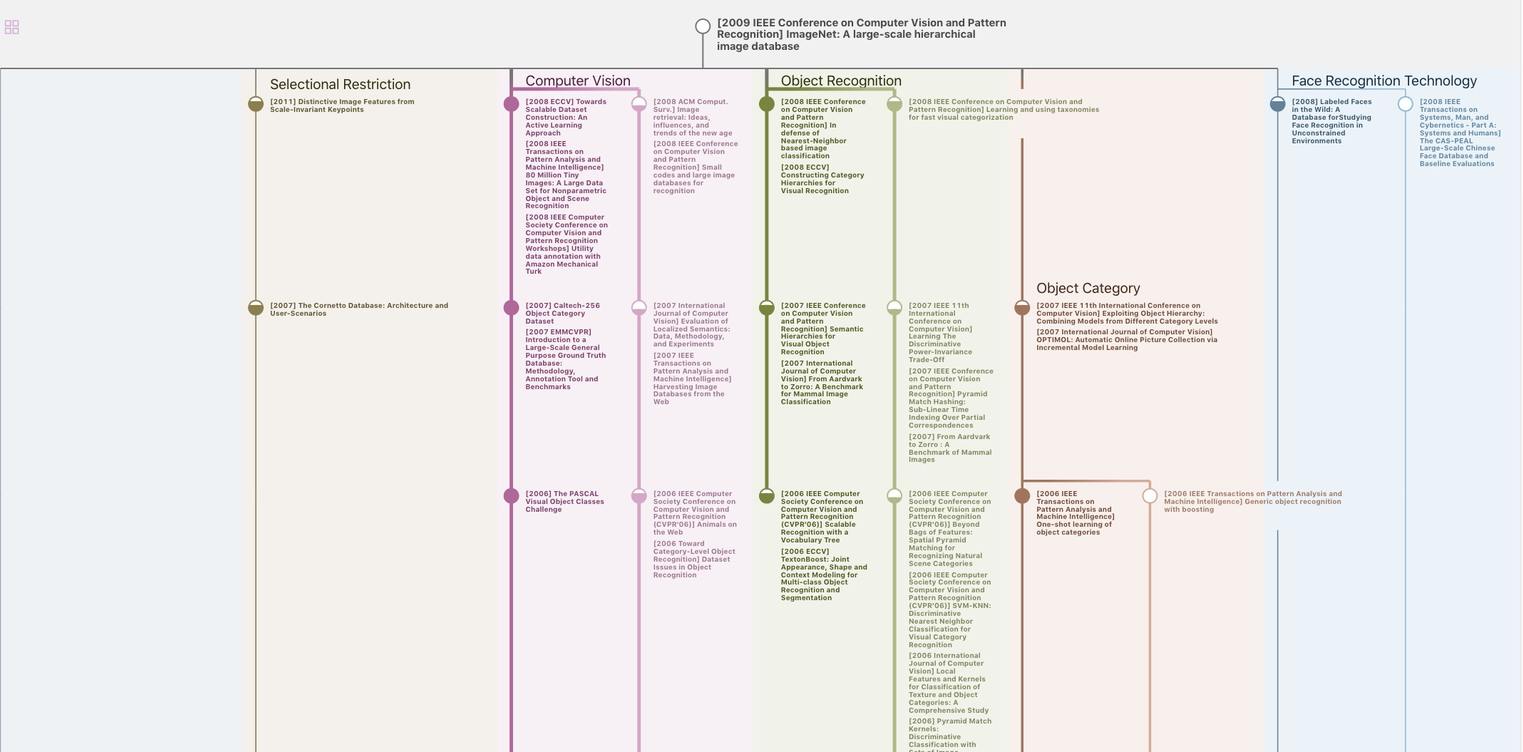
生成溯源树,研究论文发展脉络
Chat Paper
正在生成论文摘要