Weak Supervision and Clustering-Based Sample Selection for Clinical Named Entity Recognition
MACHINE LEARNING AND KNOWLEDGE DISCOVERY IN DATABASES: APPLIED DATA SCIENCE AND DEMO TRACK, ECML PKDD 2023, PT VI(2023)
摘要
One of the central tasks of medical text analysis is to extract and structure meaningful information from plain-text clinical documents. Named Entity Recognition (NER) is a sub-task of information extraction that involves identifying predefined entities from unstructured free text. Notably, NER models require large amounts of human-labeled data to train, but human annotation is costly and laborious and often requires medical training. Here, we aim to overcome the shortage of manually annotated data by introducing a training scheme for NER models that uses an existing medical ontology to assign weak labels to entities and provides enhanced domain-specific model adaptation with in-domain continual pre-training. Due to limited human annotation resources, we develop a specific module to collect a more representative test dataset from the data lake than a random selection. To validate our framework, we invite clinicians to annotate the test set. In this way, we construct two Finnish medical NER datasets based on clinical records retrieved from a hospital's data lake and evaluate the effectiveness of the proposed methods. The code is available at https://github.com/VRCMF/HAM-net.git.
更多查看译文
关键词
Named Entity Recognition,Distant Supervision,Sample Selection,Clinical Reports
AI 理解论文
溯源树
样例
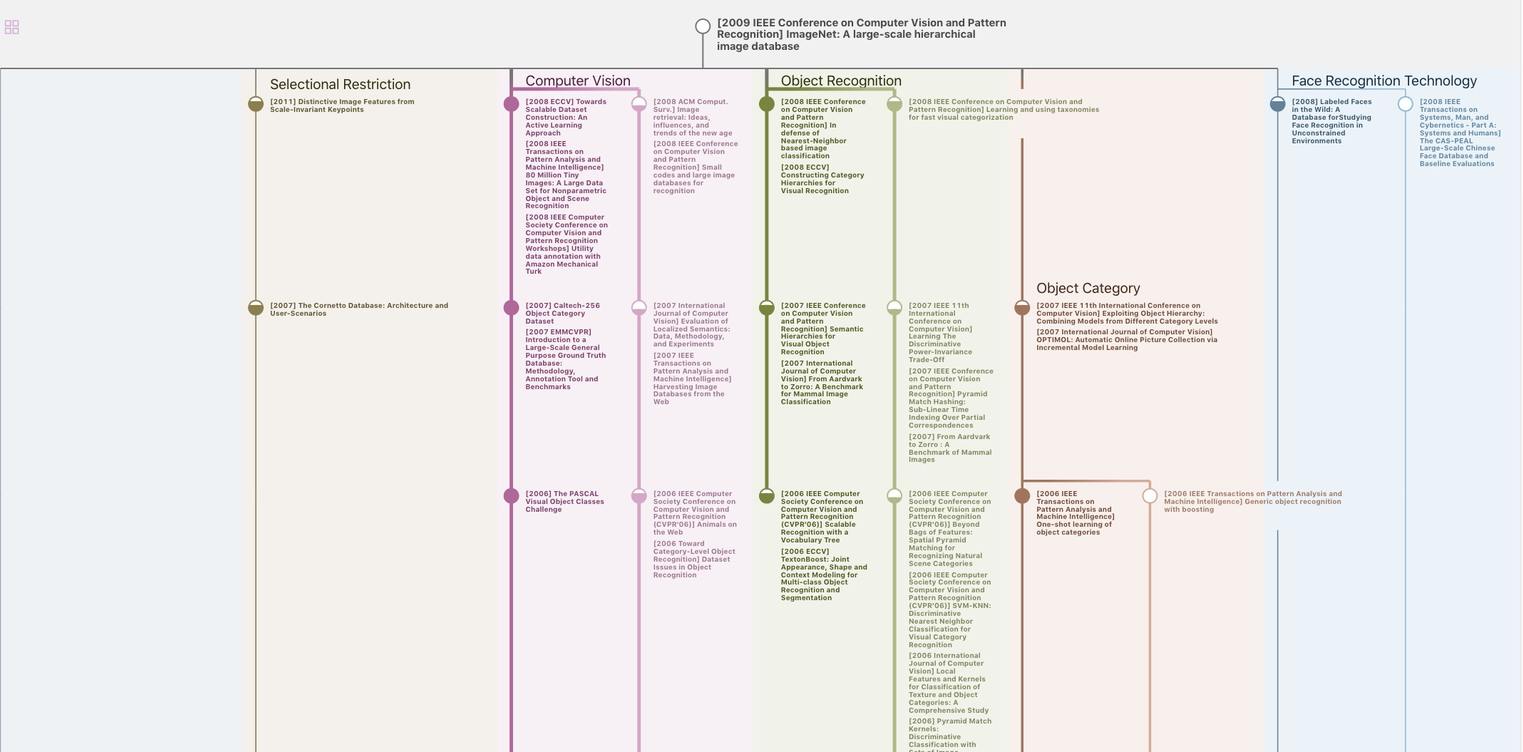
生成溯源树,研究论文发展脉络
Chat Paper
正在生成论文摘要