Transfer Robustness to Downstream Tasks Through Sampling Adversarial Perturbations
LatinX in AI at Computer Vision and Pattern Recognition Conference 2023(2023)
摘要
Due to the vulnerability of deep neural networks to adversarial attacks, adversarial robustness has grown to be a crucial problem in deep learning. Recent research has demonstrated that even small perturbations to the input data can have a large impact on the model’s output, exposing them susceptible to malicious attacks. In this work, we propose Delta Data Augmentation (DDA), a data augmentation method for enhancing transfer robustness by sampling extracted perturbations from trained models against adversarial attacks. The main idea of our work is to generate adversarial perturbations and to apply them to downstream datasets in a data augmentation fashion. Here we demonstrate, through extensive experimentation the advantages of our data augmentation method over the current State-of-the-Art in Fast Gradient Sign Method (FGSM) and Projected Gradient Descent (PGD) attacks for CIFAR10 dataset.
更多查看译文
AI 理解论文
溯源树
样例
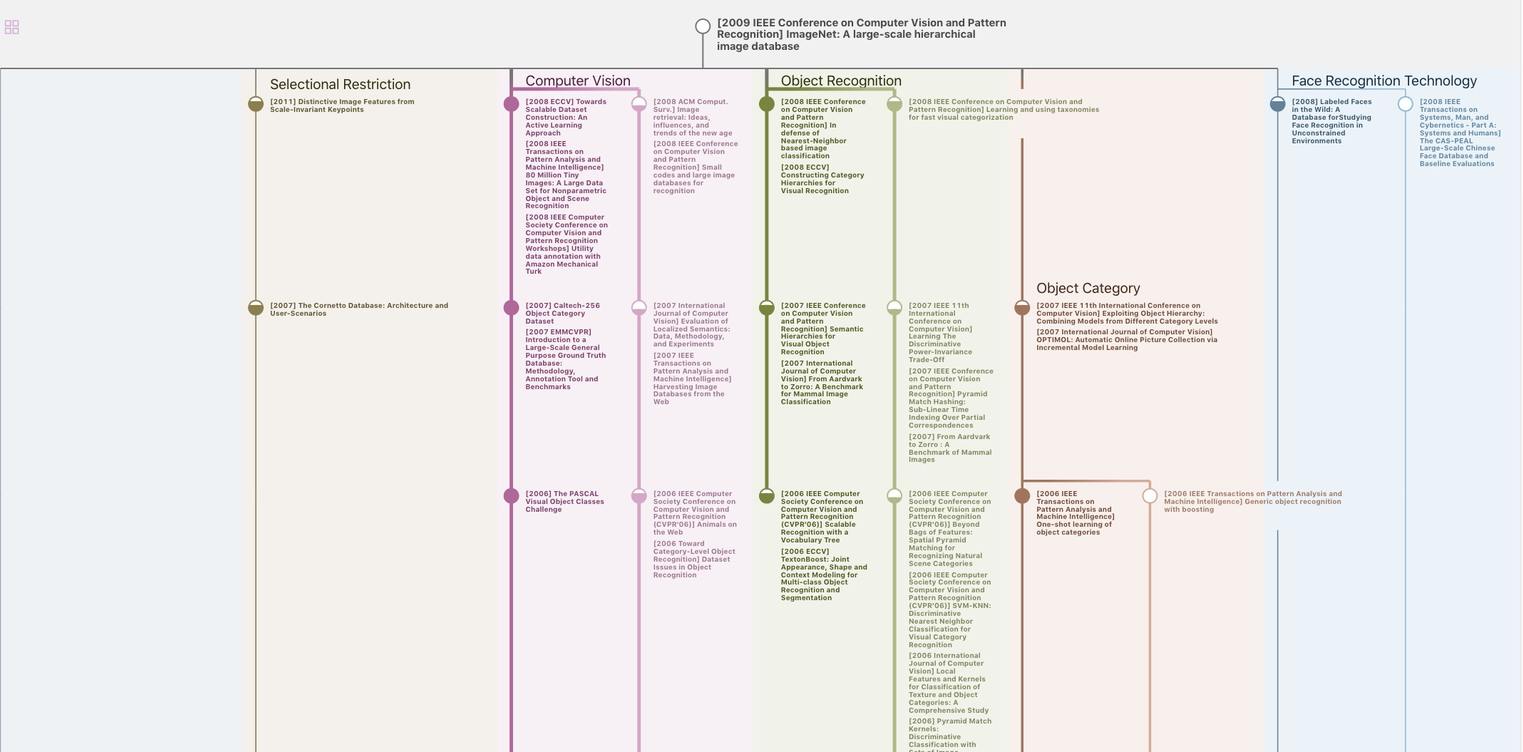
生成溯源树,研究论文发展脉络
Chat Paper
正在生成论文摘要