193MO Development of a deep learning model using a large real-world database to predict overall survival in patients with metastatic breast cancer (MBC)
ESMO Open(2023)
摘要
Despite recent improvements in MBC management, sensitivity to treatment and disease outcomes remain heterogeneous. We developed a deep learning and user-friendly model to predict individual outcomes and select the most adequate treatments according to the course of the disease. We used data from the Unicancer large national multicenter real-world Epidemiological Strategy and Medical Economics (ESME) database (NCT03275311) that included 27,855 women diagnosed between 2008 and 2020 with MBC. The primary outcome was overall survival (OS). To measure this outcome and follow the disease, treatment line (TL) initiation dates were used as essential time points, allowing us to build a dynamic deep learning survival model. The main structure of this model is the extension of a Long-Short Term Memory cell, a recurrent neural network designed to handle sequential data with irregular timing. The time-dependent concordance index (C-index) and the Integrated Brier Score (IBS) allowed the selection of the best models. The cohort included 4,857 patients with triple-negative (TN), 5,027 with HER2+ and 17,971 with hormonal receptor positive (HR+)/HER2- MBC. Median follow-up was 65.1 months (95%CI 63.8, 66.4). The areas under the receiving operator characteristics (AUCs) for 6-month and 1-year OS prediction, the sensitivity at 90% specificity for 6-month OS prediction, as well as the global C-index and IBS of the model per subtype, averaged across 5-fold cross-validation cohorts, are reported in the table for the three first TLs.Table: 193MOAverage metrics for our model per subtype for the three first TLsSubtypeInitial date: TL initiationAUC for 6-month OSSensitivity at 90% specificity for 6-month OSAUC for 1-year OSGlobal C-indexGlobal IBSTNTL10.760.400.760.690.14TNTL20.760.380.760.690.10TNTL30.750.400.760.690.08HER2+TL10.800.440.790.720.16HER2+TL20.780.390.780.710.17HER2+TL30.770.390.760.700.16HR+/HER2-TL10.810.480.790.700.16HR+/HER2-TL20.780.420.770.700.16HR+/HER2-TL30.780.420.770.710.14 Open table in a new tab Our models showed promising results in predicting OS for MBC patients of each subtype at different time points. The ability to anticipate early failure and death despite adjusted standard treatment is important for identifying patients requiring a more innovative investigation. The strong prognostic capacity of our deep learning survival model could inform treatment selection for MBC patients.
更多查看译文
关键词
deep learning model,metastatic breast cancer,breast cancer,real-world
AI 理解论文
溯源树
样例
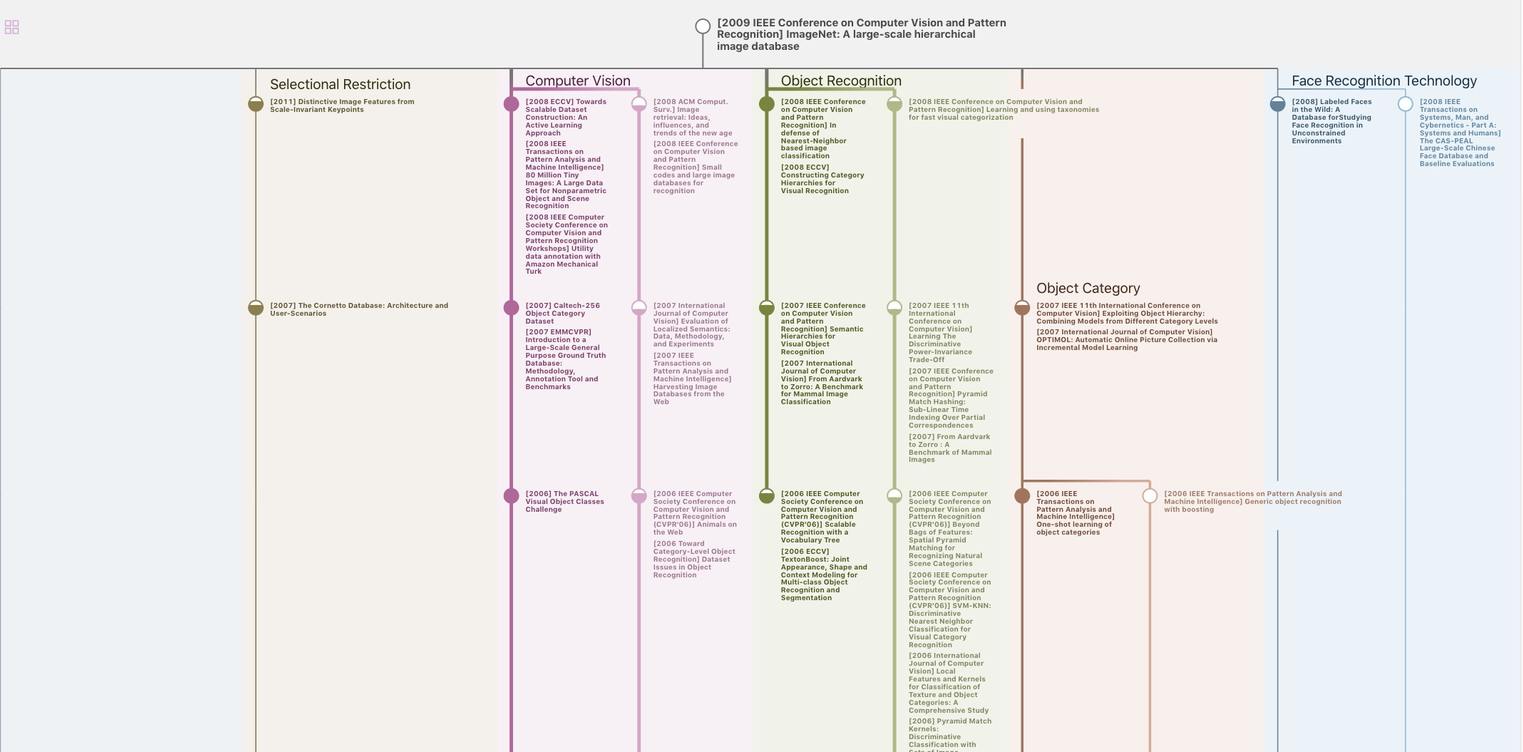
生成溯源树,研究论文发展脉络
Chat Paper
正在生成论文摘要