Expanding inclusion criteria for active surveillance in intermediate-risk prostate cancer: a machine learning approach
World journal of urology(2023)
摘要
Purpose To develop new selection criteria for active surveillance (AS) in intermediate-risk (IR) prostate cancer (PCa) patients. Methods Retrospective study including patients from 14 referral centers who underwent pre-biopsy mpMRI, image-guided biopsies and radical prostatectomy. The cohort included biopsy-naive IR PCa patients who met the following inclusion criteria: Gleason Grade Group (GGG) 1–2, PSA < 20 ng/mL, and cT1-cT2 tumors. We relied on a recursive machine learning partitioning algorithm developed to predict adverse pathological features (i.e., ≥ pT3a and/or pN + and/or GGG ≥ 3). Results A total of 594 patients with IR PCa were included, of whom 220 (37%) had adverse features. PI-RADS score (weight:0.726), PSA density (weight:0.158), and clinical T stage (weight:0.116) were selected as the most informative risk factors to classify patients according to their risk of adverse features, leading to the creation of five risk clusters. The adverse feature rates for cluster #1 (PI-RADS ≤ 3 and PSA density < 0.15), cluster #2 (PI-RADS 4 and PSA density < 0.15), cluster #3 (PI-RADS 1–4 and PSA density ≥ 0.15), cluster #4 (normal DRE and PI-RADS 5), and cluster #5 (abnormal DRE and PI-RADS 5) were 11.8, 27.9, 37.3, 42.7, and 65.1%, respectively. Compared with the current inclusion criteria, extending the AS criteria to clusters #1 + #2 or #1 + #2 + #3 would increase the number of eligible patients (+ 60 and + 253%, respectively) without increasing the risk of adverse pathological features. Conclusions The newly developed model has the potential to expand the number of patients eligible for AS without compromising oncologic outcomes. Prospective validation is warranted.
更多查看译文
关键词
Active surveillance,Intermediate risk,Machine learning,Oncological outcomes,Prostate cancer
AI 理解论文
溯源树
样例
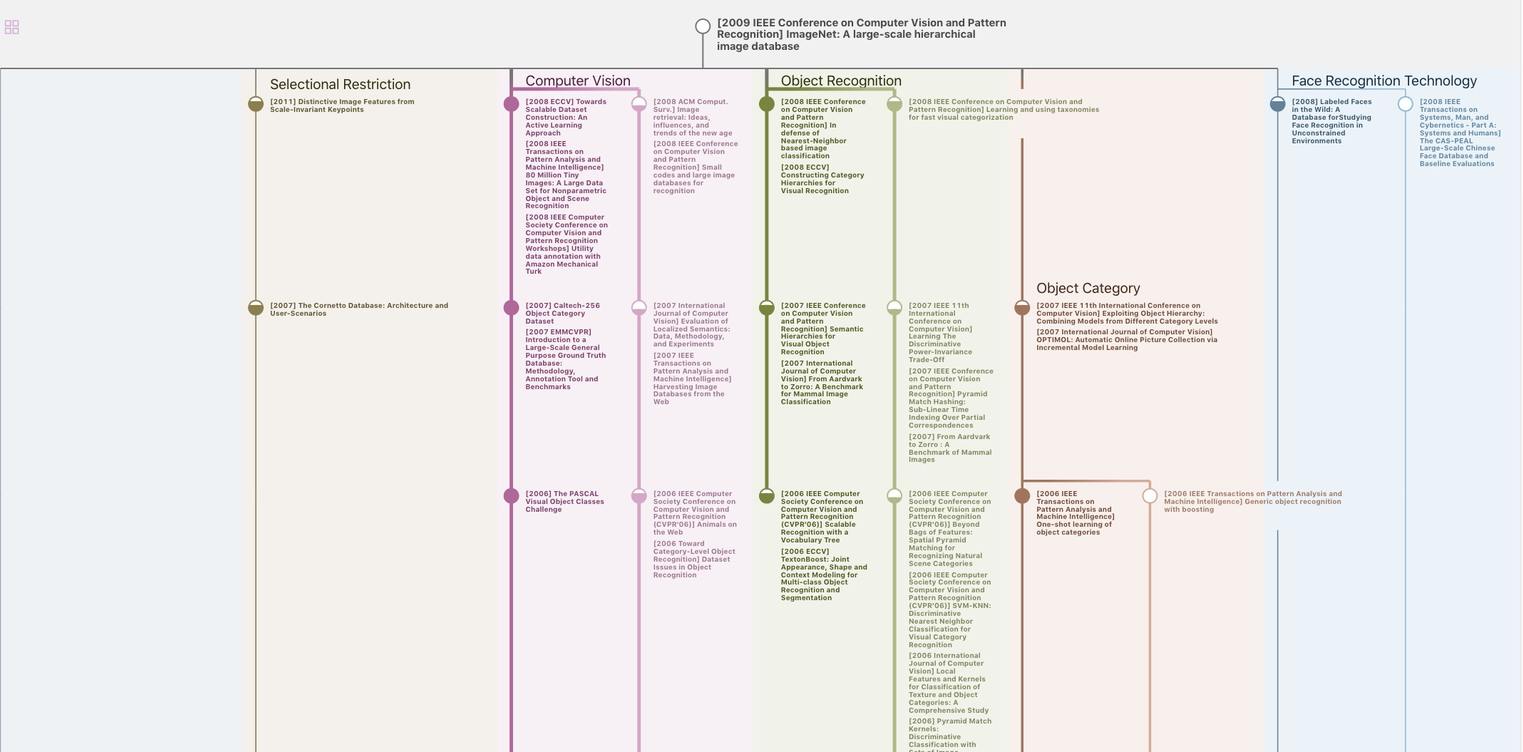
生成溯源树,研究论文发展脉络
Chat Paper
正在生成论文摘要