Learning interpretable causal networks from very large datasets, application to 400,000 medical records of breast cancer patients
iScience(2024)
摘要
Discovering causal effects is at the core of scientific investigation but remains challenging when only observational data is available. In practice, causal networks are difficult to learn and interpret, and limited to relatively small datasets. We report a more reliable and scalable causal discovery method (iMIIC), based on a general mutual information supremum principle, which greatly improves the precision of inferred causal relations while distinguishing genuine causes from putative and latent causal effects. We showcase iMIIC on synthetic and real-world healthcare data from 396,179 breast cancer patients from the US Surveillance, Epidemiology, and End Results program. More than 90% of predicted causal effects appear correct, while the remaining unexpected direct and indirect causal effects can be interpreted in terms of diagnostic procedures, therapeutic timing, patient preference or socio-economic disparity. iMIIC’s unique capabilities open up new avenues to discover reliable and interpretable causal networks across a range of research fields.
更多查看译文
关键词
Cancer systems biology,Computing methodology,Health informatics,Machine learning
AI 理解论文
溯源树
样例
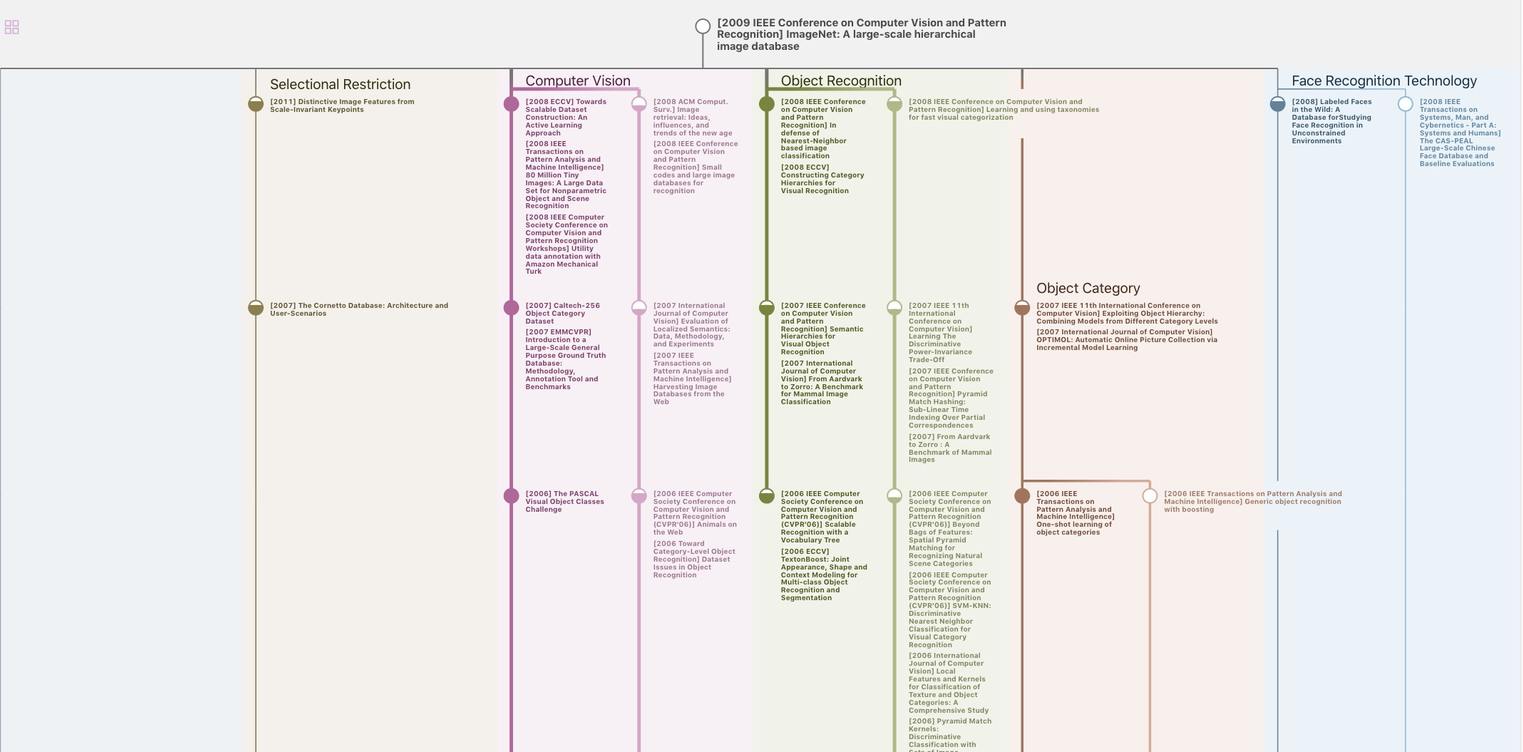
生成溯源树,研究论文发展脉络
Chat Paper
正在生成论文摘要