A joint model of the individual mean and within-subject variability of a longitudinal outcome with a competing risks time-to-event outcome
arxiv(2023)
摘要
Motivated by recent findings that within-subject (WS) variability of longitudinal biomarkers is a risk factor for many health outcomes, this paper introduces and studies a new joint model of a longitudinal biomarker with heterogeneous WS variability and competing risks time-to-event outcome. Specifically, our joint model consists of a linear mixed-effects multiple location-scale submodel for the individual mean trajectory and WS variability of the longitudinal biomarker and a semiparametric cause-specific Cox proportional hazards submodel for the competing risks survival outcome. The submodels are linked together via shared random effects. We derive an expectation-maximization (EM) algorithm for semiparametric maximum likelihood estimation and a profile-likelihood method for standard error estimation. We implement scalable computational algorithms that can scale to biobank-scale data with tens of thousands of subjects. Our simulation results demonstrate that the proposed method has superior performance and that classical joint models with homogeneous WS variability can suffer from estimation bias, invalid inference, and poor prediction accuracy in the presence of heterogeneous WS variability. An application of the developed method to the large Multi-Ethnic Study of Atherosclerosis (MESA) data not only revealed that subject-specific WS variability in systolic blood pressure (SBP) is highly predictive of heart failure and death, but also yielded more accurate dynamic prediction of heart failure or death by accounting for both the mean trajectory and WS variability of SBP. Our user-friendly R package \textbf{JMH} is publicly available at \url{https://github.com/shanpengli/JMH}.
更多查看译文
关键词
longitudinal outcome,risks,variability,within-subject,time-to-event
AI 理解论文
溯源树
样例
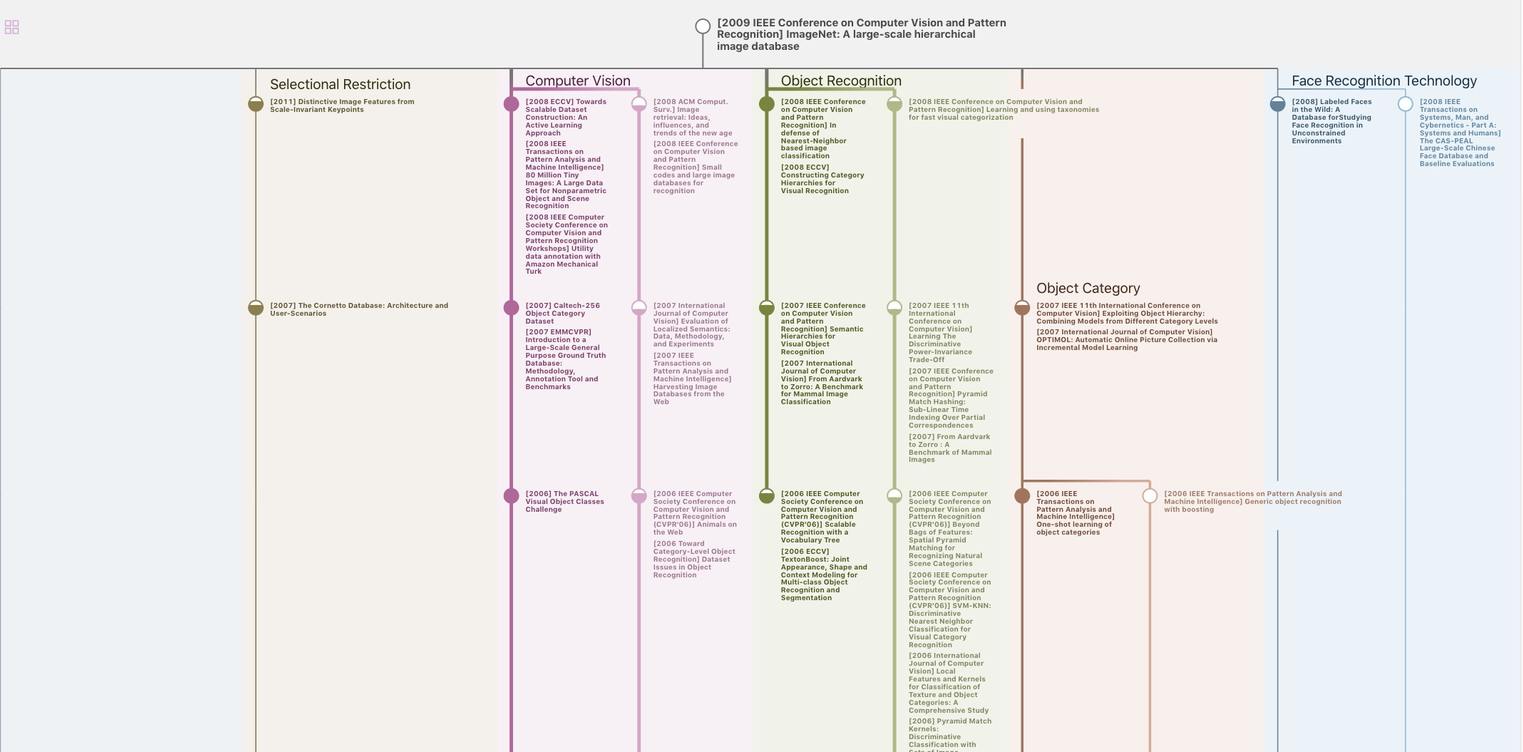
生成溯源树,研究论文发展脉络
Chat Paper
正在生成论文摘要