Accuracy Prediction for NAS Acceleration using Feature Selection and Extrapolation
arxiv(2022)
摘要
Predicting the accuracy of candidate neural architectures is an important capability of NAS-based solutions. When a candidate architecture has properties that are similar to other known architectures, the prediction task is rather straightforward using off-the-shelf regression algorithms. However, when a candidate architecture lies outside of the known space of architectures, a regression model has to perform extrapolated predictions, which is not only a challenging task, but also technically impossible using the most popular regression algorithm families, which are based on decision trees. In this work, we are trying to address two problems. The first one is improving regression accuracy using feature selection, whereas the other one is the evaluation of regression algorithms on extrapolating accuracy prediction tasks. We extend the NAAP-440 dataset with new tabular features and introduce NAAP-440e, which we use for evaluation. We observe a dramatic improvement from the old baseline, namely, the new baseline requires 3x shorter training processes of candidate architectures, while maintaining the same mean-absolute-error and achieving almost 2x fewer monotonicity violations, compared to the old baseline's best reported performance. The extended dataset and code used in the study have been made public in the NAAP-440 repository.
更多查看译文
关键词
nas acceleration,feature selection,prediction,accuracy
AI 理解论文
溯源树
样例
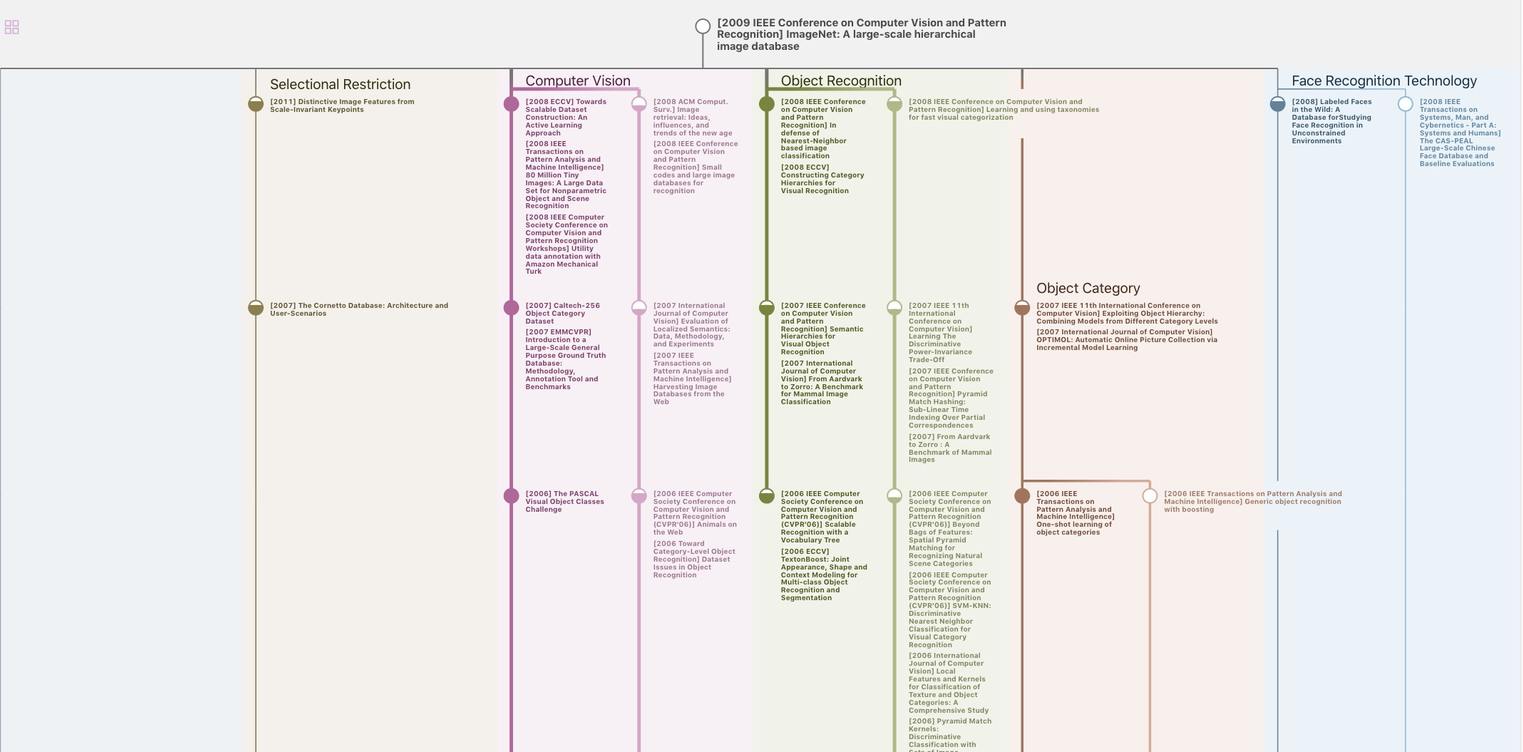
生成溯源树,研究论文发展脉络
Chat Paper
正在生成论文摘要