Robust Moderately Clipped LASSO for Simultaneous Outlier Detection and Variable Selection
SANKHYA-SERIES B-APPLIED AND INTERDISCIPLINARY STATISTICS(2022)
摘要
Outlier detection has become an important and challenging issue in high-dimensional data analysis due to the coexistence of data contamination and high-dimensionality. Most existing widely used penalized least squares methods are sensitive to outliers due to the l 2 loss. In this paper, we proposed a Robust Moderately Clipped LASSO (RMCL) estimator, that performs simultaneous outlier detection, variable selection and robust estimation. The RMCL estimator can be efficiently solved using the coordinate descent algorithm in a convex-concave procedure. Our numerical studies demonstrate that the RMCL estimator possesses superiority in both variable selection and outlier detection and thus can be advantageous in difficult prediction problems with data contamination.
更多查看译文
关键词
Outlier detection, Variable selection, Robust regression, High-dimensional data, MCL, Convex-concave
AI 理解论文
溯源树
样例
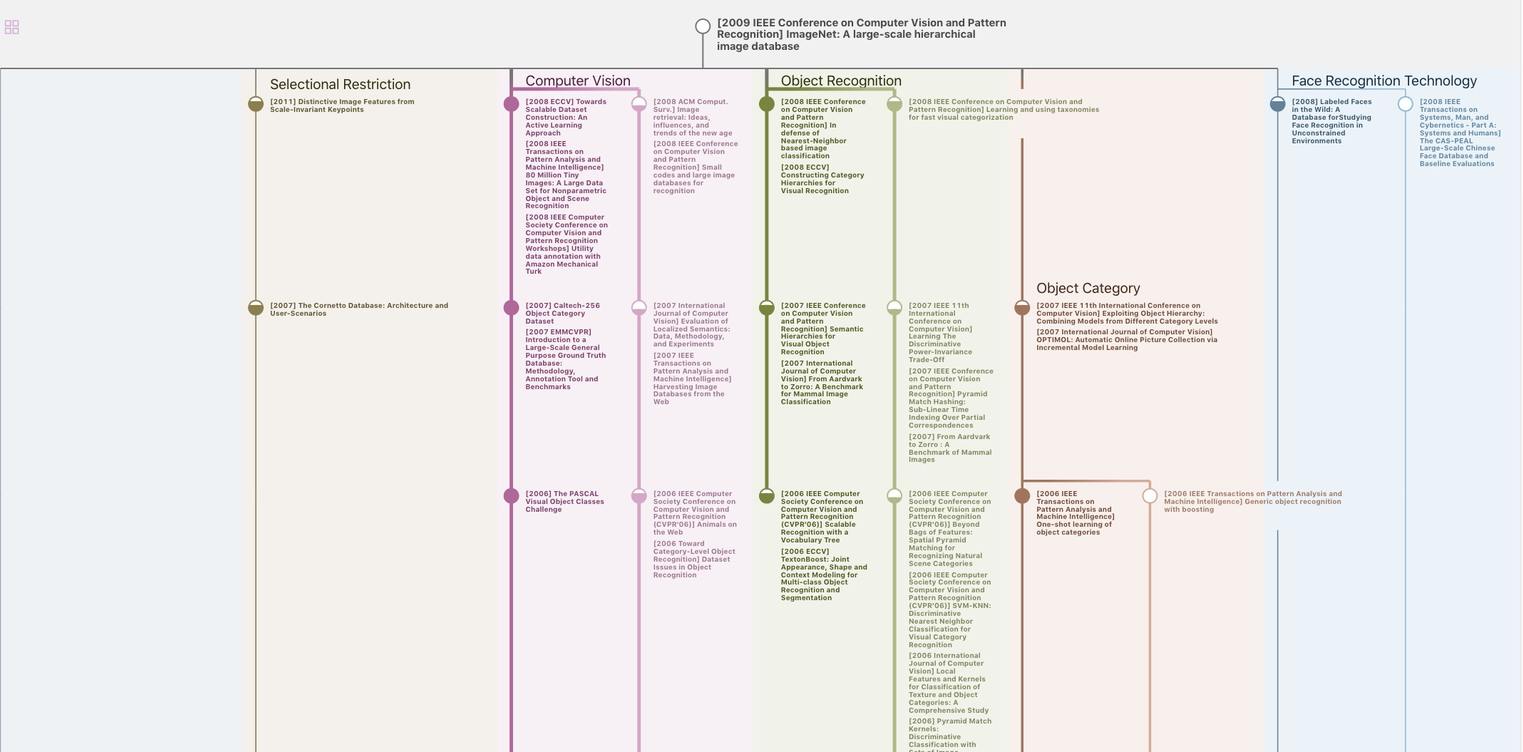
生成溯源树,研究论文发展脉络
Chat Paper
正在生成论文摘要