Action recognition by key trajectories
Pattern Analysis and Applications(2022)
摘要
Human action recognition is an active field of research that intends to explain what a subject is doing in an input video. Deep learning architectures serve as the foundation for cutting-edge approaches. Recent research, on the other hand, indicates that hand-crafted characteristics are complementary and, when combined, can enhance classification accuracy. Cutting-edge approaches are based on deep learning architectures. Recent research, however, indicates that hand-crafted features complement each other and can help boost classification accuracy when combined. We introduce the key trajectories approach that is based on the popular, hand-crafted method, improved dense trajectories. Our work explores how pose estimation can be used to find meaningful key points to reduce computational time, undesired noise, and to guarantee a stable frame processing rate. Furthermore, we tested how feature-tracking behaves with dense inverse search and with a frame to frame subject key point estimation. Our proposal was tested on the KTH and UCF11 datasets employing Bag-of-words and on the UCF50 and HMDB datasets using Fisher Vector, where we got an accuracy performance of 95.71, 84.88, 92.9, and 81.3%, respectively. Also, our proposal can recognize subject actions in video eight times faster compared to its dense counterpart. To maximize the bag-of-words classification performance, we illustrate how the hyperparameters affect both accuracy and computation time. Precisely, we present an exploration of the vocabulary size, the SVM hyperparameter, the descriptor’s distinctiveness, and the subject body key points.
更多查看译文
关键词
Action recognition,Pose estimation,Dense trajectories,Key trajectories
AI 理解论文
溯源树
样例
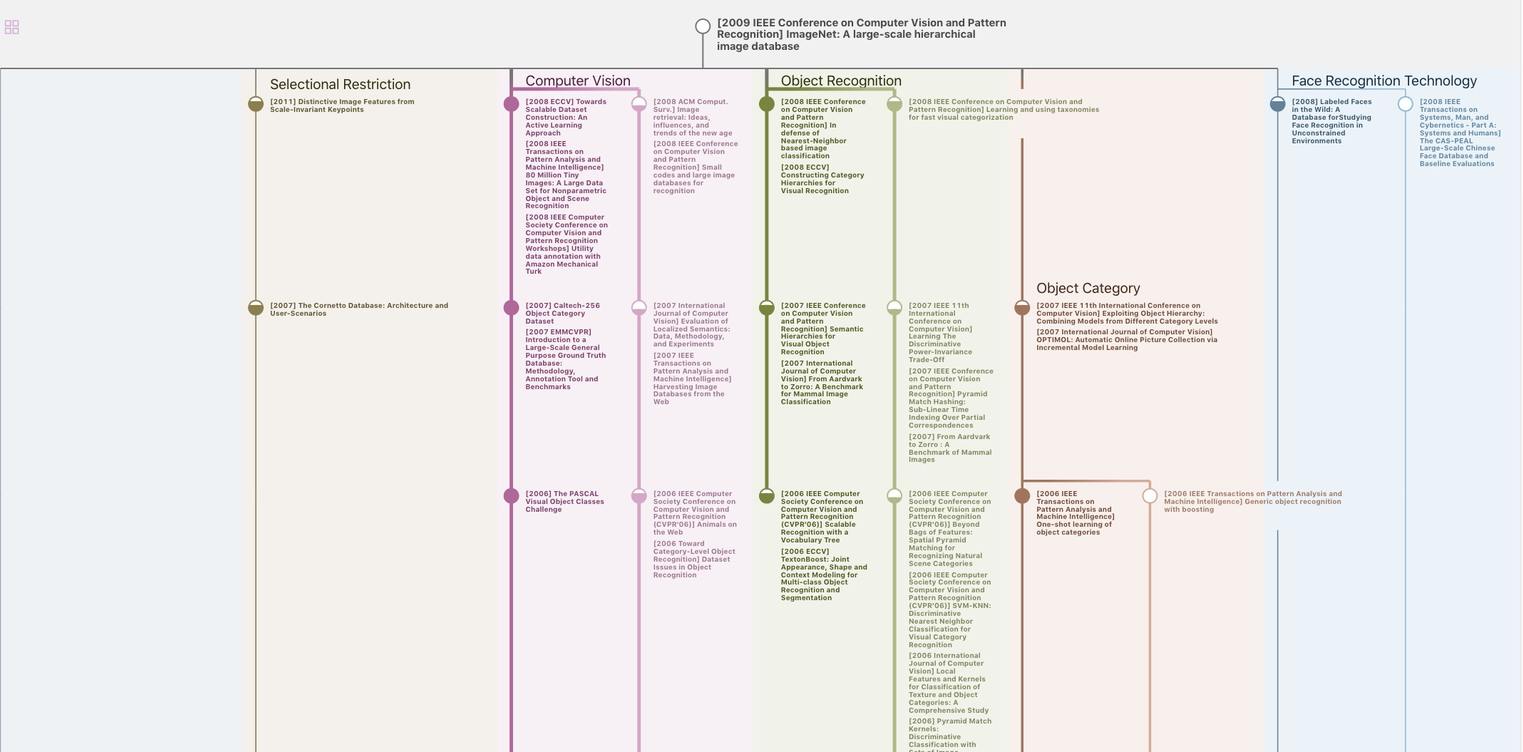
生成溯源树,研究论文发展脉络
Chat Paper
正在生成论文摘要