Deep Learning for Automated Normal Liver Volume Estimation
RADIOLOGY(2022)
摘要
HomeRadiologyVol. 302, No. 2 PreviousNext Reviews and CommentaryFree AccessEditorialDeep Learning for Automated Normal Liver Volume EstimationJacob Sosna Jacob Sosna Author AffiliationsFrom the Department of Radiology, Hadassah Medical Center, Faculty of Medicine, Hebrew University of Jerusalem, Jerusalem, Israel 91120.Address correspondence to the author (e-mail: [email protected]).Jacob Sosna Published Online:Oct 26 2021https://doi.org/10.1148/radiol.2021212010MoreSectionsPDF ToolsImage ViewerAdd to favoritesCiteTrack CitationsPermissionsReprints ShareShare onFacebookTwitterLinked In See also the article by Perez et al in this issue.Dr Sosna is professor and chairman of the imaging division at Hadassah Hebrew University Medical Center in Jerusalem, Israel. He also serves as vice dean in the Medical Faculty of the Hebrew University. Dr Sosna’s research focuses on advancing clinical applications in cutting-edge CT technology, advanced imaging visualization tools, and quantitative analysis of image characteristics in oncologic patients.Download as PowerPointOpen in Image Viewer Advanced CT-based liver analysis is a key task in many clinical applications. The analysis may include liver contour and volume estimation, blood vessel identification, and, if tumors are present, tumor detection and characterization. Clinical applications include hepatomegaly and liver cirrhosis assessment, hepatic volumetry, hepatic transplant planning, liver regeneration after hepatectomy, evaluation and planning for resection liver surgery, and monitoring of liver metastases, among many others (1,2). The liver analysis must be accurate, robust, and fast so that the radiologist can perform it routinely. CT-based liver analysis is known to be a very challenging task. The main difficulties include the ambiguity of the liver boundary, the complexity of the liver surface, the presence of surrounding organs, the contrast variability between liver parenchyma and vessels, the different tumor sizes and shapes, and the presence of many small metastases (3).Hepatomegaly is defined as abnormal enlargement of the liver that can result from a wide variety of causes, including inflammatory, infectious, neoplastic, and congenital, as well as other conditions. Physical examination is classically used for assessment of the enlarged liver but is unreliable. Imaging diagnosis of hepatomegaly can be carried out with use of US, MRI, or CT with linear craniocaudal measurements. The liver has a complex three-dimensional structure, and therefore, a single measurement may not reflect its true size. A single linear measurement may underestimate or overestimate the liver size with normal variants, such as a Riedel lobe, or with changes in the size of segment I or left lobe relative hypertrophy.Volumetric assessment provides an accurate and reliable measure of the size of the liver but classically requires manual delineation of the liver contours, a time-consuming and error-prone procedure seldom performed in clinical practice. Various methods for the semiautomatic and automatic segmentation of the liver have been developed (4). Artificial intelligence (AI)–based liver segmentation and volume assessment can be automated, potentially offering a more practical approach.To determine liver volume and establish thresholds for hepatomegaly, in a study reported in this issue of Radiology, Perez et al (5) used a validated deep learning AI tool that automatically segmented the liver. Liver volumes were derived from patients who underwent multidetector CT for colorectal screening with CT colonography (1960 patients) or renal donor evaluation (contrast-enhanced CT, 1105 patients) at a single medical center. The performance of the craniocaudal and maximal three-dimensional linear measures was assessed. The mean standardized automated liver volume ± standard deviation was 1533 mL ± 375, with a normal distribution. Patient weight was the major determinant of liver volume and demonstrated a linear relationship. A linear weight-based upper limit of normal hepatomegaly threshold was thus derived. A craniocaudal threshold of 19 cm was only 71% sensitive and 86% specific for hepatomegaly, and a maximal three-dimensional linear threshold of 24 cm was 78% sensitive and 66% specific. In a subset of 189 patients in whom the deep learning tool and the semiautomated or manual method were compared, the median difference in hepatic volume was only 2.3% (38 mL).This study provides a basis for the definition of the normal size of the liver in a population, but is it representative of the real distribution of the liver size? Different populations may have different distributions of liver sizes, as was assessed in evaluation of live potential liver donors (6,7). Further evaluation may be needed to assess the validity of the results for larger and more diverse populations. The ability to use deep learning in different populations may make this task much easier.The use of automatic computerized tools may be appealing for estimation of the liver volume. Classic delineation is a tedious approach when performed manually. AI-based methods enable a reliable and automatic analysis and are a potential solution to ensure liver identification and selection and to obtain accurate and precise comprehensive volumetric measurements instead of partial, approximate linear ones. The strength of the article by Perez et al is the use of CT studies that were not intended for the evaluation of the liver (CT colonography scans, CT scans in renal donors). In such cases, the reading radiologist usually does not assess the morphologic characteristics and size of the liver.Are we at a point where our reading paradigm might change? Currently, we read and report on all available information in the study that is related to the clinical question, as well as incidental findings that might be clinically relevant. We can envision a comprehensive assessment tool that would include evaluation of all solid organs. At this stage, a comprehensive analytic tool using AI for all organs is not available. If AI is used for the assessment of liver volume or spleen or kidney volume at every CT examination, should we generate a second report that would include this information? Whether this information is of clinical value is still not yet known. Potentially, we could find changes in organ volume in serial imaging studies before they become clinically important, such as in patients with cirrhosis.The use of AI may be also intended for nonclinical purposes. In population-based imaging, AI algorithms may analyze all CT scans obtained at every institution and gather information that could be used for definition of normal values of multiple parameters, including the one that was evaluated in the study by Perez and colleagues.How should we define normal limits? Are these the values between the upper and lower limits of a parameter in a sample of the normal population: the normal range? Most often, it is assumed that the parameter in question among the population will follow a Gaussian distribution (8). Usually, the interval of two standard deviations from the mean is used as the definition of normal values for a normally distributed continuous variable. While appealing in its simplicity and elegance, this approach carries some limitations. What should be done with patients below or above the normal limits? Should patients incidentally found with large or small livers undergo further evaluation? This might find some underlining pathologic anomaly but may also just add cost and anxiety to the patient with no clinically relevant abnormality.The study by Perez et al carries some limitations. It mainly includes the question of clinical implication of the results. Although the strength is the sample size, there is still missing information on the subset of patients with large or small livers. Are these mainly a reflection of normal variations, or do they represent cases with true underlying disease? Further validation is required, especially in terms of its application to more diverse cohorts that include diseased livers or patients with systemic diseases. The use of the technique may also be problematic for cases with benign or malignant lesions or with underlying parenchymal abnormality. This would need to be assessed separately, as the algorithm used may not be optimal in diseased livers and would need to be adjusted for these purposes.AI is currently mainly narrowly oriented for a specific task in a specific population (eg, locating suspected lung nodules at oncologic follow-up). The applicability of AI tools as shown in the study by Perez et al for assessing a large population for rather simple tasks (eg, liver volume) has an important potential to widen the scope of our profession. It can be used for data mining of parameters currently not assessed in clinical practice that might increase our understanding of population health and further define imaging markers that would improve our ability as radiologists to provide more imaging-based information and for the clinicians to improve patient care.Disclosures of Conflicts of Interest: J.S. Grants from Philips; consulting fees from High-rad; former president of the Israel Radiological Society; consultant to the Editor for Radiology and co-chair of the mentored reviewer program.References1. Luo S, Li X, Li J. Review on the methods of automatic liver segmentation from abdominal images. J Comput Commun 2014;02(02):1–7. Crossref, Google Scholar2. Gotra A, Sivakumaran L, Chartrand G, et al. Liver segmentation: indications, techniques and future directions. Insights Imaging 2017;8(4):377–392. Crossref, Medline, Google Scholar3. Freiman M, Eliassaf O, Taieb Y, Joskowicz L, Sosna J. A bayesian approach for liver analysis: algorithm and validation study. Med Image Comput Comput Assist Interv 2008;11(Pt 1):85–92. Medline, Google Scholar4. Moghbel M, Mashohor S, Mahmud R, et al. Review of liver segmentation and computer assisted detection/diagnosis methods in computed tomography. Artif Intell Rev 2018;50(4):497–537. Crossref, Google Scholar5. Perez AA, Noe-Kim V, Lubner MG, et al. Deep learning CT-based quantitative visualization tool for liver volume estimation: defining normal and hepatomegaly. Radiology 2021. https://doi.org/10.1148/radiol.2021210531. Published online October 26, 2021. Google Scholar6. Yu HC, You H, Lee H, Jin ZW, Moon JI, Cho BH. Estimation of standard liver volume for liver transplantation in the Korean population. Liver Transpl 2004;10(6):779–783. Crossref, Medline, Google Scholar7. Kapileshwer V, Naidu CS, Godara R, Rao P, Sharma S, Vijayvergia V. Standard liver volume estimation in Indian population: Need for an accurate formula. Ind J Transplant 2013;7(2):39–41. Crossref, Google Scholar8. Flachskampf FA, Nihoyannopoulos P. Our obsession with normal values. Echo Res Pract 2018;5(2):R17–R21. Crossref, Medline, Google ScholarArticle HistoryReceived: Aug 8 2021Revision requested: Aug 20 2021Revision received: Aug 23 2021Accepted: Aug 26 2021Published online: Oct 26 2021Published in print: Feb 2022 FiguresReferencesRelatedDetailsAccompanying This ArticleDeep Learning CT-based Quantitative Visualization Tool for Liver Volume Estimation: Defining Normal and HepatomegalyOct 26 2021RadiologyRecommended Articles Deep Learning CT-based Quantitative Visualization Tool for Liver Volume Estimation: Defining Normal and HepatomegalyRadiology2021Volume: 302Issue: 2pp. 336-342Personalized Reference Intervals Will Soon Become Standard in Radiology ReportsRadiology2021Volume: 301Issue: 2pp. 348-349Liver Surgery: Important Considerations for Pre- and Postoperative ImagingRadioGraphics2022Volume: 42Issue: 3pp. 722-740Liver Surface Nodularity Score Allows Prediction of Cirrhosis Decompensation and DeathRadiology2016Volume: 283Issue: 3pp. 711-722Combination of Active Transfer Learning and Natural Language Processing to Improve Liver Volumetry Using Surrogate Metrics with Deep LearningRadiology: Artificial Intelligence2019Volume: 1Issue: 1See More RSNA Education Exhibits Artificial Intelligence and Liver Segmentation: Current Applications and Future DirectionsDigital Posters2019Imaging Assessment after Partial Hepatectomies: Surgical Technique and Type of Parenchymal Transection Performed: Normal Findings and ComplicationsDigital Posters2022Associating Liver Partition and Portal Vein Ligation for Staged Hepatectomy in Hepatocellular Carcinoma Treatment: What Radiologists Need to KnowDigital Posters2019 RSNA Case Collection Biliary Hamartomas in Cirrhotic LiverRSNA Case Collection2021Riedel's lobeRSNA Case Collection2021Caroli SyndromeRSNA Case Collection2020 Vol. 302, No. 2 Metrics Altmetric Score PDF download
更多查看译文
关键词
normal liver,deep learning,volume
AI 理解论文
溯源树
样例
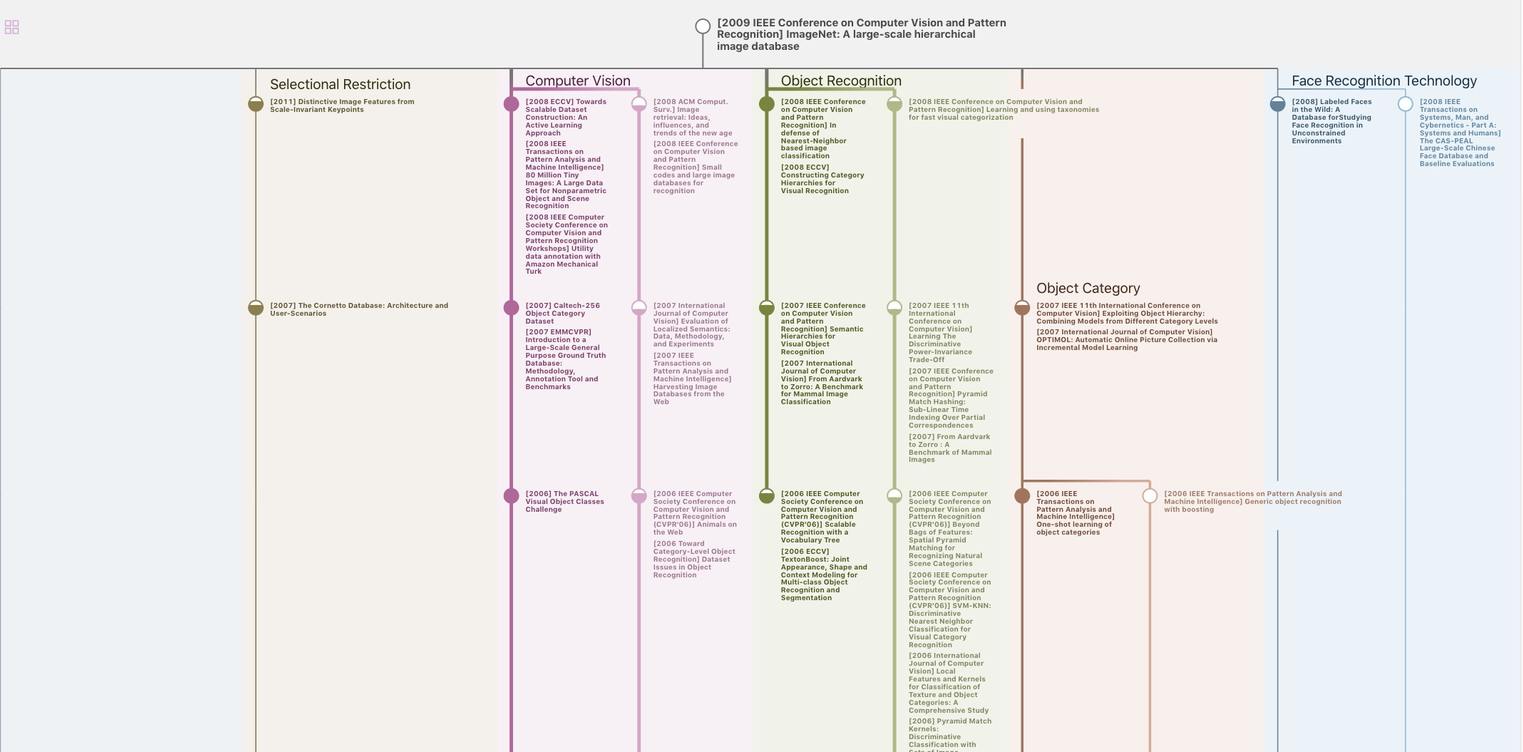
生成溯源树,研究论文发展脉络
Chat Paper
正在生成论文摘要