Assessing YOLACT plus plus for real time and robust instance segmentation of medical instruments in endoscopic procedures
2021 43RD ANNUAL INTERNATIONAL CONFERENCE OF THE IEEE ENGINEERING IN MEDICINE & BIOLOGY SOCIETY (EMBC)(2021)
摘要
Image-based tracking of laparoscopic instruments plays a fundamental role in computer and robotic-assisted surgeries by aiding surgeons and increasing patient safety. Computer vision contests, such as the Robust Medical Instrument Segmentation (ROBUST-MIS) Challenge, seek to encourage the development of robust models for such purposes, providing large, diverse, and high-quality datasets. To date, most of the existing models for instance segmentation of medical instruments were based on two-stage detectors, which provide robust results but are nowhere near to the real-time, running at 5 frames-per-second (fps) at most. However, for the method to be clinically applicable, a real-time capability is utmost required along with high accuracy. In this paper, we propose the addition of attention mechanisms to the YOLACT architecture to allow real-time instance segmentation of instruments with improved accuracy on the ROBUST-MIS dataset. Our proposed approach achieves competitive performance compared to the winner of the 2019 ROBUST-MIS challenge in terms of robustness scores, obtaining 0.313 MI_DSC and 0338 MI_NSD while reaching real-time performance at >45 fps.
更多查看译文
关键词
Deep learning, laparoscopy, segmentation, surgical instrument, real-time
AI 理解论文
溯源树
样例
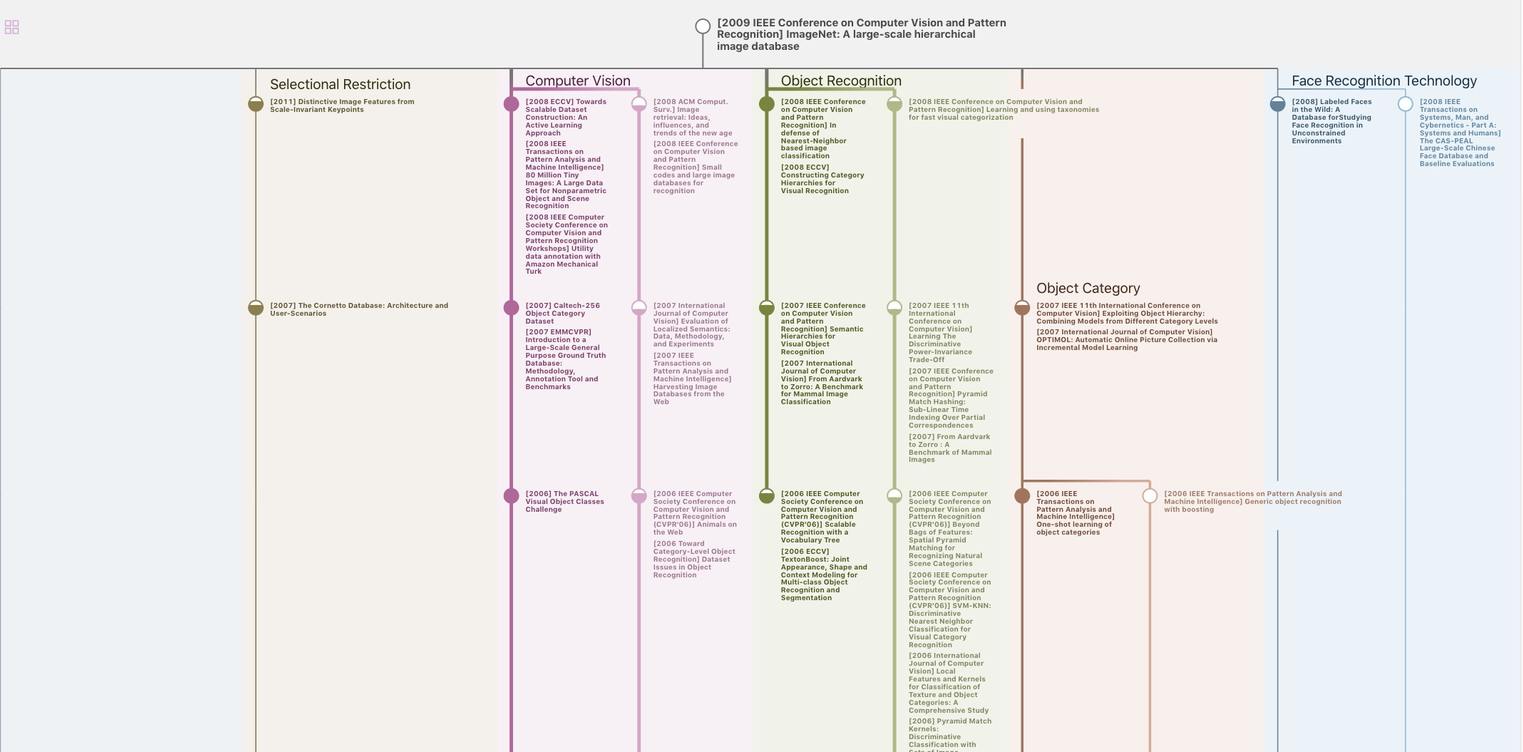
生成溯源树,研究论文发展脉络
Chat Paper
正在生成论文摘要