On the Use of Neural Networks with Censored Time-to-Event Data.
ISMCO(2020)
摘要
Motivation: The objective of this work is to confront artificial neural network models with time-to-event data, using specific ways to handle censored observations such as pseudo-observations and tailored loss functions. Methods: Different neural network models were compared. Cox-CC (Kvamme et al., 2019) uses a loss function based on a case-control approximation. DeepHit (Lee et al., 2019) is a model that estimates the probability mass function and combines log-likelihood with a ranking loss. DNNSurv (Zhao et al., 2019) circumvents the problem of censoring by using pseudo-observations. We also proposed other ways of computing pseudo-observations. We investigated the prediction ability of these models using data simulated from an Accelerated Failure Time model (Friedman et al., 2001), with different censoring rates. We simulated 100 data sets of 4,000 samples and 20 variables each, with pairwise interactions and non-linear effects of random subsets of these variables. Models were compared using the concordance index and integrated Brier score. We applied the methods to the METABRIC breast cancer data set, including 1,960 patients, 6 clinical covariates and the expression of 863 genes. Results: In the simulation study, we obtained the highest c-indices and lower integrated Brier score with CoxTime for low censoring and pseudo-discrete with high censoring. On the METABRIC data, the neural networks obtained comparable 5-year and 10-year discrimination performances with slightly higher values for the models based on optimised pseudo-observations. Availability: https://github.com/eroblin/NN_Pseudobs
更多查看译文
关键词
Survival data, Neural networks, Pseudo-observations
AI 理解论文
溯源树
样例
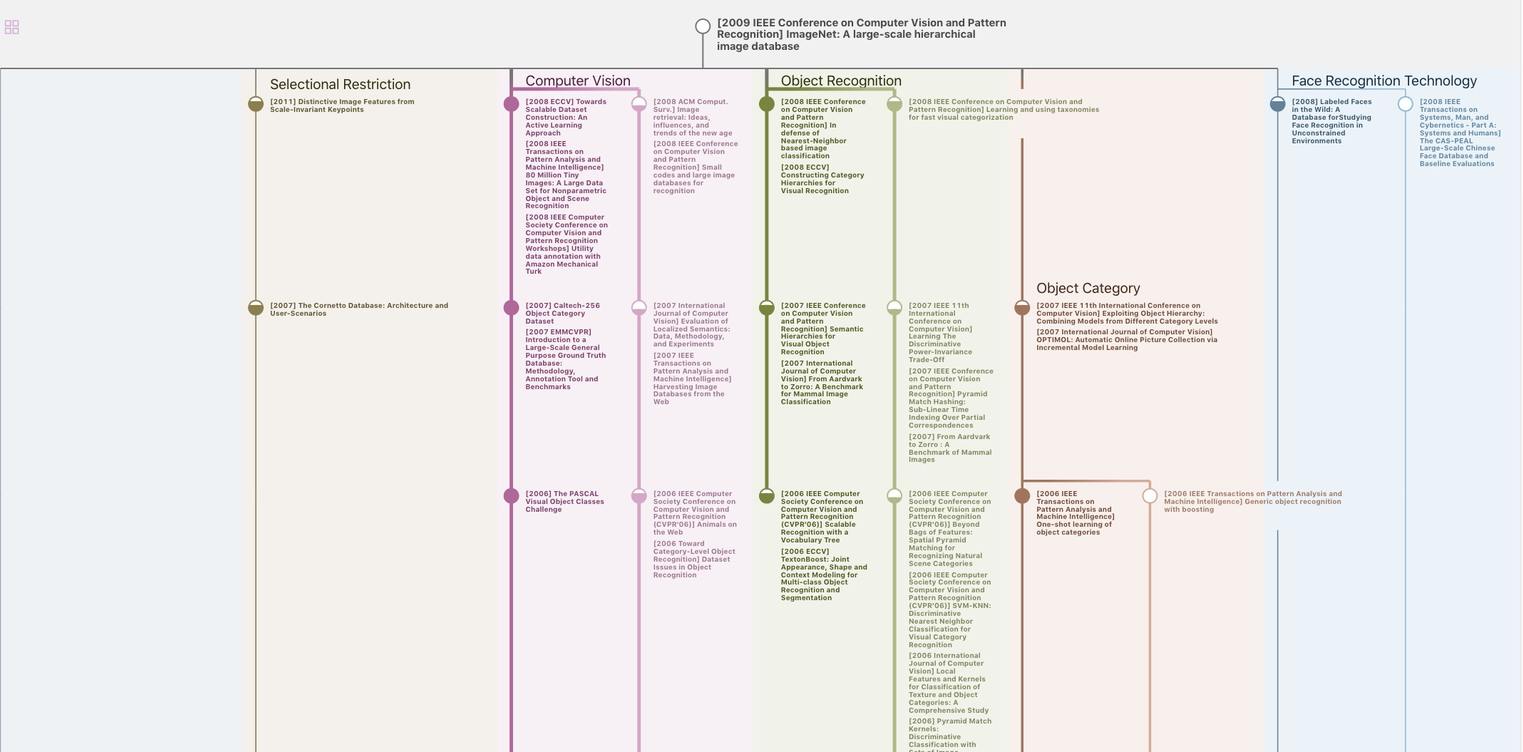
生成溯源树,研究论文发展脉络
Chat Paper
正在生成论文摘要