Improving Enzyme Optimum Temperature Prediction with Resampling Strategies and Ensemble Learning.
JOURNAL OF CHEMICAL INFORMATION AND MODELING(2020)
摘要
Accurate prediction of the optimal catalytic temperature (T-opt) of enzymes is vital in biotechnology, as enzymes with high T-opt values are desired for enhanced reaction rates. Recently, a machine learning method (temperature optima for microorganisms and enzymes, TOME) for predicting Topt was developed. TOME was trained on a normally distributed data set with a median T-opt of 37 degrees C and less than 5% of T-opt values above 85 degrees C, limiting the method's predictive capabilities for thermostable enzymes. Due to the distribution of the training data, the mean squared error on T-opt values greater than 85 degrees C is nearly an order of magnitude higher than the error on values between 30 and 50 degrees C. In this study, we apply ensemble learning and resampling strategies that tackle the data imbalance to significantly decrease the error on high T-opt values (>85 degrees C) by 60% and increase the overall R-2 value from 0.527 to 0.632. The revised method, temperature optima for enzymes with resampling (TOMER), and the resampling strategies applied in this work are freely available to other researchers as Python packages on GitHub.
更多查看译文
关键词
optimum temperature prediction,ensemble learning,resampling strategies
AI 理解论文
溯源树
样例
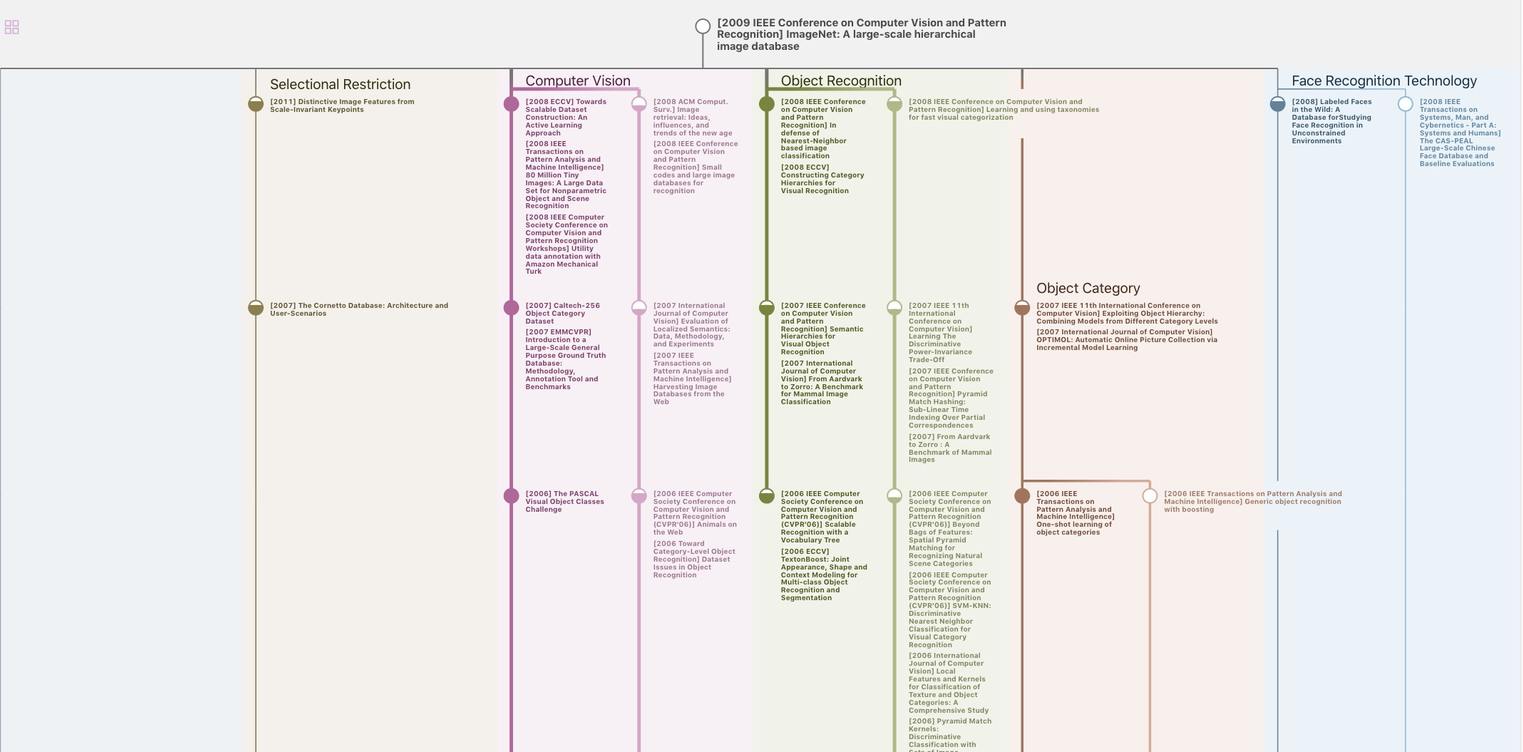
生成溯源树,研究论文发展脉络
Chat Paper
正在生成论文摘要