Nonlinear Regression Operating on Microstructures Described from Topological Data Analysis for the Real-Time Prediction of Effective Properties.
MATERIALS(2020)
摘要
Real-time decision making needs evaluating quantities of interest (QoI) in almost real time. When these QoI are related to models based on physics, the use of Model Order Reduction techniques allows speeding-up calculations, enabling fast and accurate evaluations. To accommodate real-time constraints, a valuable route consists of computing parametric solutions-the so-called computational vademecums-that constructed off-line, can be inspected on-line. However, when dealing with shapes and topologies (complex or rich microstructures) their parametric description constitutes a major difficulty. In this paper, we propose using Topological Data Analysis for describing those rich topologies and morphologies in a concise way, and then using the associated topological descriptions for generating accurate supervised classification and nonlinear regression, enabling an almost real-time evaluation of QoI and the associated decision making.
更多查看译文
关键词
machine learning,data-driven mechanics,TDA,Code2Vect,nonlinear regression,effective properties,microstructures
AI 理解论文
溯源树
样例
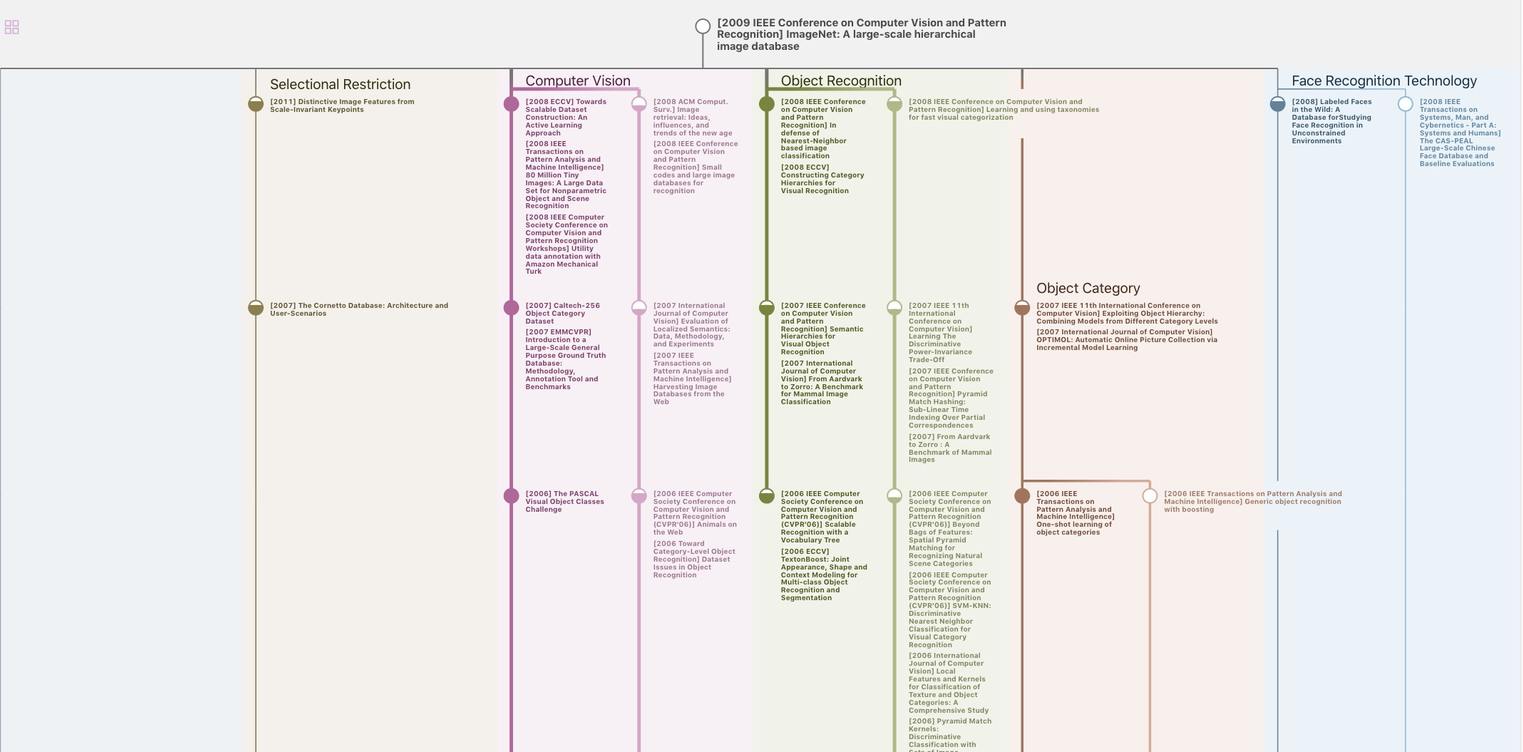
生成溯源树,研究论文发展脉络
Chat Paper
正在生成论文摘要